AlphaDDA: strategies for adjusting the playing strength of a fully
Por um escritor misterioso
Descrição
Artificial intelligence (AI) has achieved superhuman performance in board games such as Go, chess, and Othello (Reversi). In other words, the AI system surpasses the level of a strong human expert player in such games. In this context, it is difficult for a human player to enjoy playing the games with the AI. To keep human players entertained and immersed in a game, the AI is required to dynamically balance its skill with that of the human player. To address this issue, we propose AlphaDDA, an AlphaZero-based AI with dynamic difficulty adjustment (DDA). AlphaDDA consists of a deep neural network (DNN) and a Monte Carlo tree search, as in AlphaZero. AlphaDDA learns and plays a game the same way as AlphaZero, but can change its skills. AlphaDDA estimates the value of the game state from only the board state using the DNN. AlphaDDA changes a parameter dominantly controlling its skills according to the estimated value. Consequently, AlphaDDA adjusts its skills according to a game state. AlphaDDA can adjust its skill using only the state of a game without any prior knowledge regarding an opponent. In this study, AlphaDDA plays Connect4, Othello, and 6x6 Othello with other AI agents. Other AI agents are AlphaZero, Monte Carlo tree search, the minimax algorithm, and a random player. This study shows that AlphaDDA can balance its skill with that of the other AI agents, except for a random player. AlphaDDA can weaken itself according to the estimated value. However, AlphaDDA beats the random player because AlphaDDA is stronger than a random player even if AlphaDDA weakens itself to the limit. The DDA ability of AlphaDDA is based on an accurate estimation of the value from the state of a game. We believe that the AlphaDDA approach for DDA can be used for any game AI system if the DNN can accurately estimate the value of the game state and we know a parameter controlling the skills of the AI system.
Understanding Employees' Strengths Helps Aligning Them with Tasks

Average score in each game. DDA was active only in games 2 and 4.

The Psychology of Trading: Series 34 Exam Insights - FasterCapital

Difficult flow of the player, adapted from Hunicke and Chapman [7]

Total score of each game on the x axis.
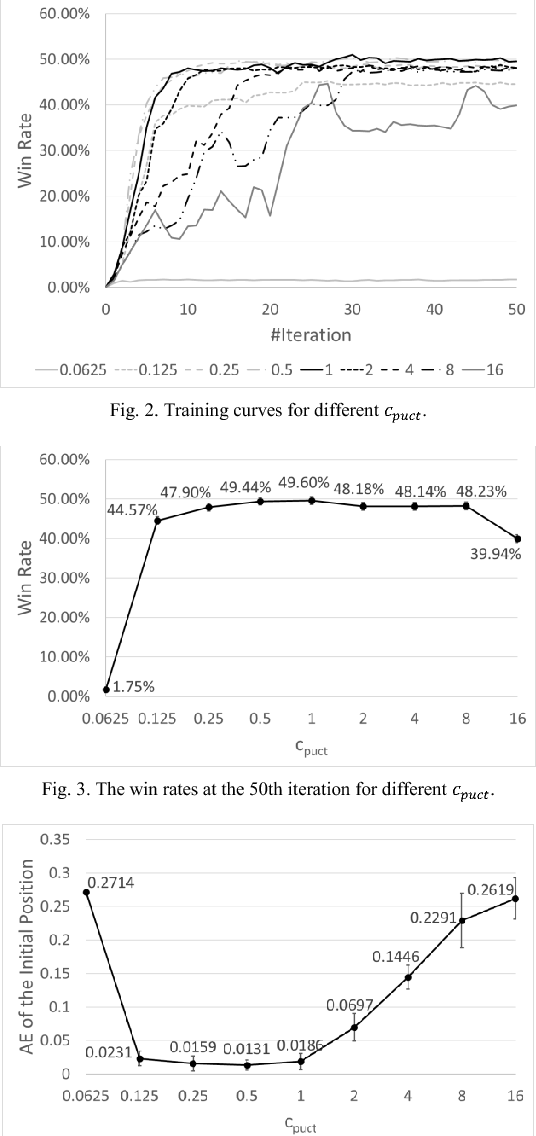
AlphaZero for a Non-Deterministic Game
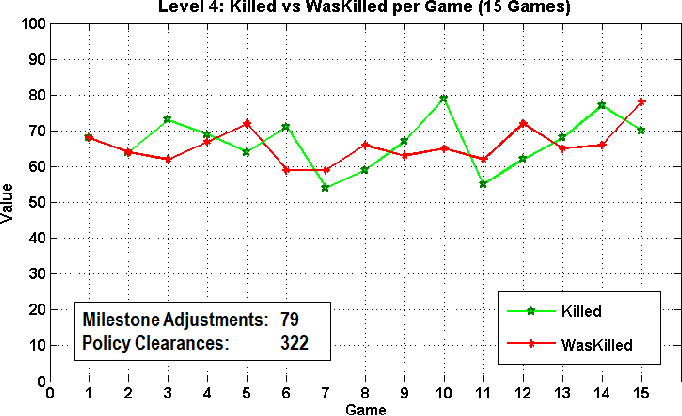
PDF] Skilled Experience Catalogue: A Skill-Balancing Mechanism for Non- Player Characters using Reinforcement Learning
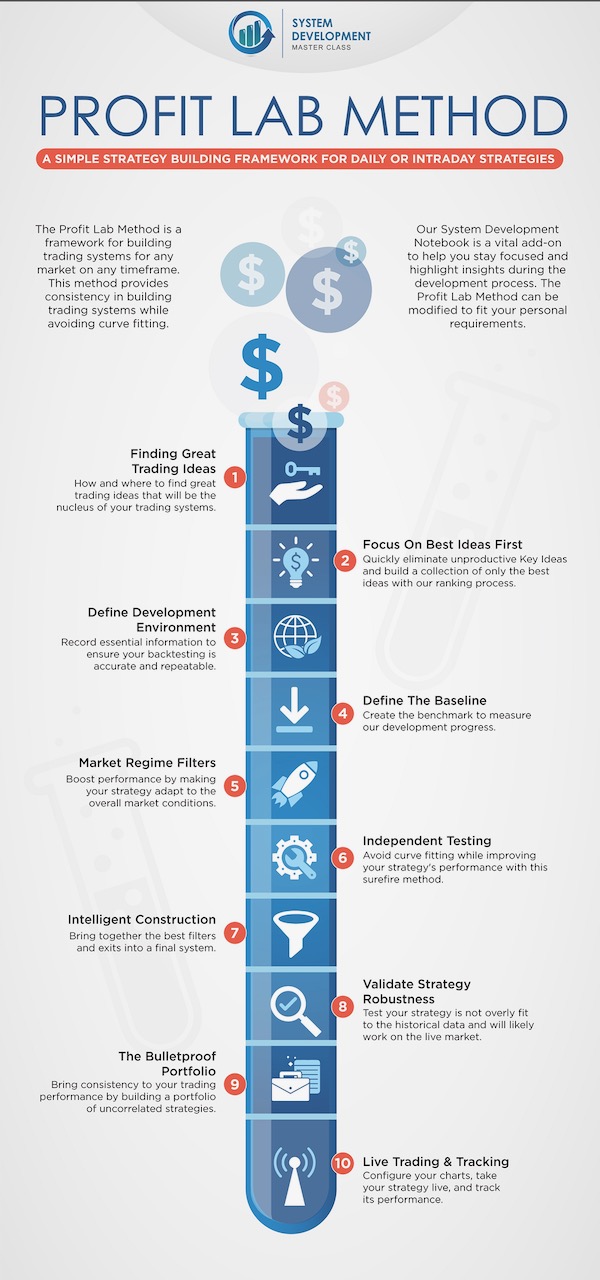
Creating Strategies Using Build Alpha - Helping you Master EasyLanguage
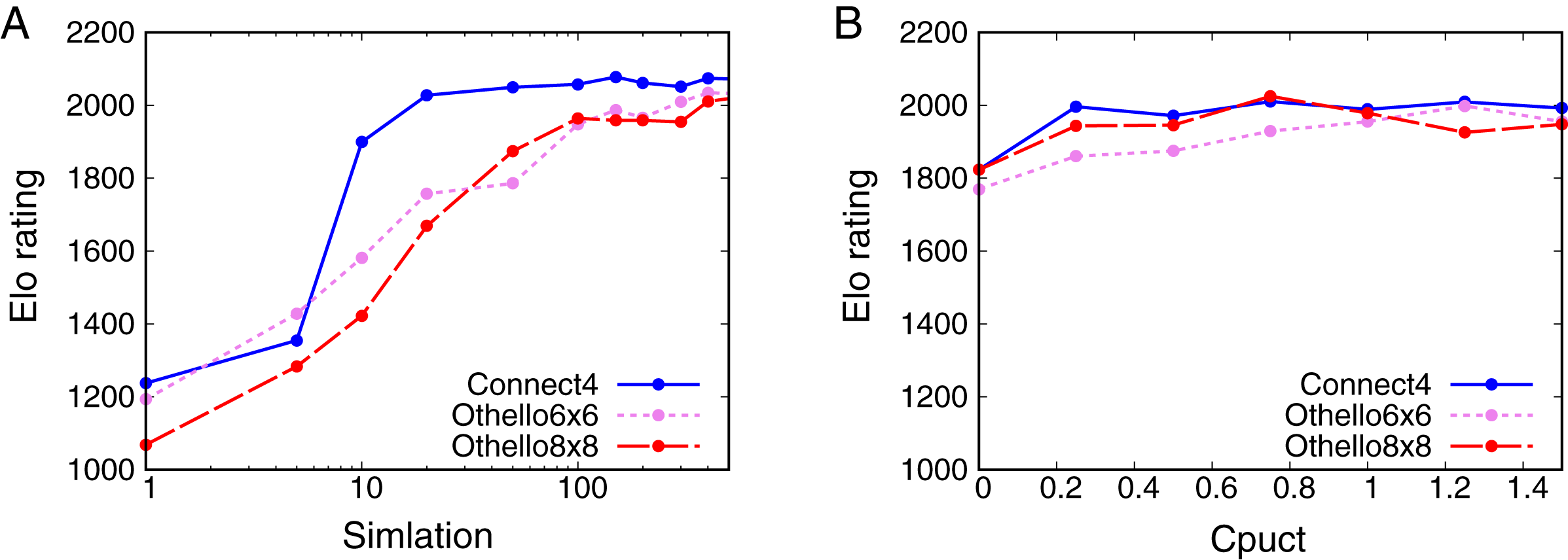
AlphaDDA: strategies for adjusting the playing strength of a fully trained AlphaZero system to a suitable human training partner [PeerJ]
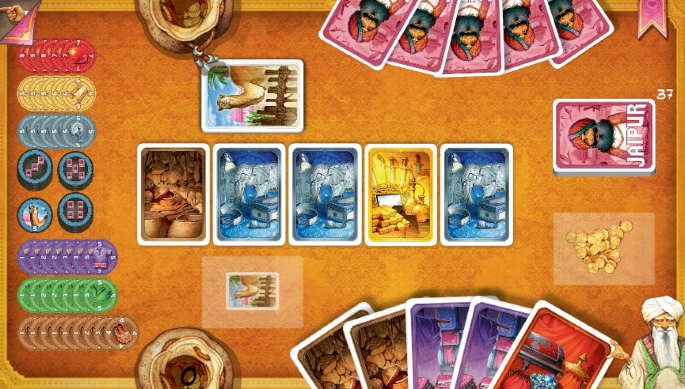
Mastering the Card Game of Jaipur Through Zero-Knowledge Self-Play Reinforcement Learning and Action Masks

arxiv-sanity
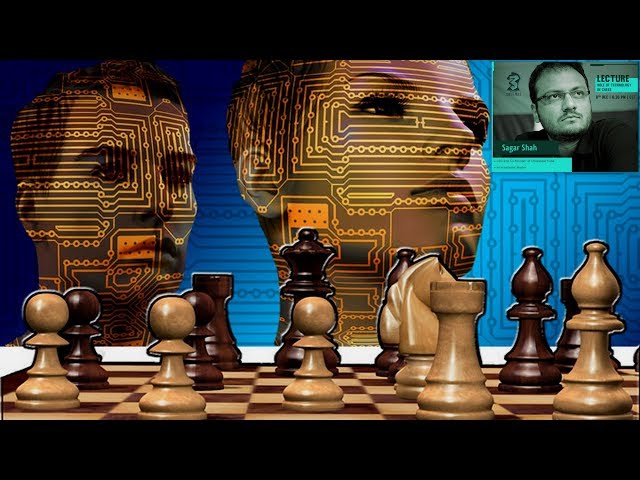
Game Changer: AlphaZero's Groundbreaking Chess Strategies and the Promise of AI
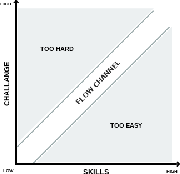
PDF] Dynamic difficulty adjustment through parameter manipulation for Space Shooter game
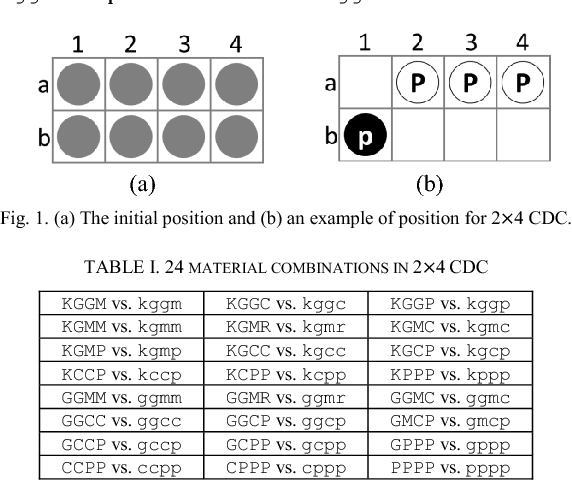
AlphaZero for a Non-Deterministic Game
de
por adulto (o preço varia de acordo com o tamanho do grupo)